I’m sure you’ve heard of “the death of the cookie” by now. It’s hard to miss. Even people outside our industry are aware that times are a-changin’.
Apple is turning privacy into a competitive advantage and is gearing up to take maximum advantage with its own advertising offering. Google and Facebook are scrambling and have rolled out solutions like Consent Mode, Enhanced Conversions and the Conversion API. Did we implement them? Sure. Did we actually see an impact? Well… No.
Even with all possible solutions implemented, measurement still looks like this.
Why? Because they’re tiny band-aids for Titanic-sized problems:
- Cookie-consent popups are massively decreasing the number of people we can track
- Every year, households own more and more internet-capable devices
- Browser Tracking Prevention caps 3rd party cookie lifetimes and blocks fingerprinting
- App Tracking Transparency decreases the ability to track customer journeys across different apps on Apple devices
- Customer Journeys are longer than the maximum conversion window settings
- Cookies have never been particularly good at measurement (read more on this in the survey we did with Ipsos)
- Offline channels like radio or TV are not included
- Internal marketing variables like promotions, product availability or price updates are not included
Some problems described above aren’t new and have always made attribution difficult. Looking beyond click-based or impression-based attribution models has always been absolutely essential for businesses with long customer journeys, or even to just properly value upper-funnel campaigns. For these hard-to-track cases, some of the go-to methods were:
- Giving monetary value to soft conversions that have a causal relationship to the hard conversion, and optimizing campaigns on these soft conversions, knowing the hard conversions will follow sooner or later
- Building holistic dashboards that don’t depend on cookie-based attribution
- Just putting common sense before DaTa DrIvEn MaRkEtIng 🙂
These methods allowed us to break free from the elevated cost-per-clicks that are considered normal at the bottom of the funnel. We could now also target a much larger addressable audience, namely everyone that hasn’t yet expressed an interest in their search and browsing behaviour. It actually allowed performance marketers to build brands, with aspirational video creatives.
Great, we were no longer playing the game of the SEA expert that can only think in binary code.
Media plans created with common sense outperform media plans that are created with just click-based attribution insights. It’s that simple. At least, when looking at the right metrics. But, there was no way to really validate this holistic approach. No way to take it to the next level, to optimize it further.
This is where Marketing Mix Modelling comes into play. MMM isn’t new. Some advertisers have been doing it for years, usually outsourcing it to specialized data science firms. It was just very costly, and in our experience, these firms don’t really understand digital channels well enough for them to be able to give good advice. They’re just very smart statisticians. We’ve seen cases for our clients for which the main recommendation of such a firm was to push more budget to branded paid search, which is just silly.
Luckily, the analytics discipline of MMM has become more accessible because of 3 big trends:
A vast open-source community has emerged and qualitative MMM frameworks are available, free of charge. Some of these frameworks are even developed by GAFA themselves.
Cloud computing has evolved to a point where the feasibility and costs involved to have a data warehouse just for media, are no longer obstacles. Python and R can also be applied at scale for data governance in the cloud.
Scientific rigorousness in our digital marketing. Our industry has matured in terms of usage of statistical processes & algorithms for media planning.
These developments made us comfortable enough to take the plunge. We decided to launch our spin-off LYKTA, a toolkit for marketing measurement. Included in the offering are:
- Data storage & management
- Business intelligence, dashboards & reports
- Marketing mix modelling, drive to web modelling, advanced AB tests
- Multi-moment strategy, consultancy
This is how it works:
MMM relies on data, obviously. The more data and the more variation within the data, the more accurate the model will be. It’s, by the way, not required to have an API connection to your platforms. Simple CSVs can go a long way. First, the key variables and drivers of online and offline sales are identified. Then, machine learning algorithms use regression analysis to forecast sales based on those drivers, resulting in the actual model. With the model, the output reports are created that attribute sales to each driver. The insights from these reports can be used to optimize online spend and marketing activities.
The examples below will clear up what is truly essential: What can I expect in terms of output?
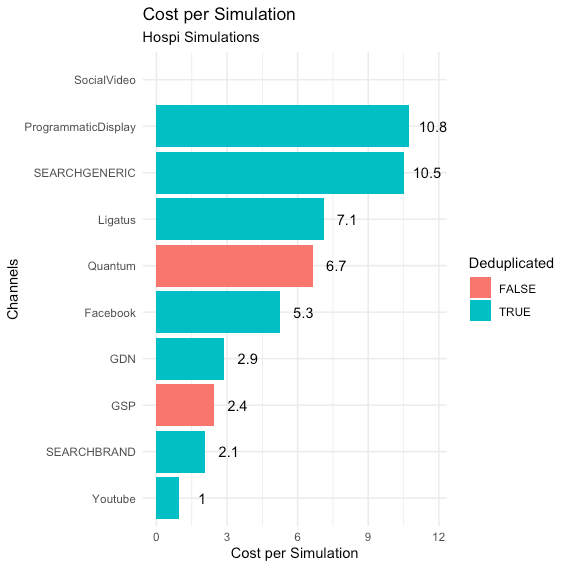
Modelled cost per soft conversion. YouTube has the lowest, which isn’t something you usually see. It’s because we don’t just look at the conversions coming from website visits that resulted from clicks on the ad, but instead, modelled the full impact of the campaign with MMM.
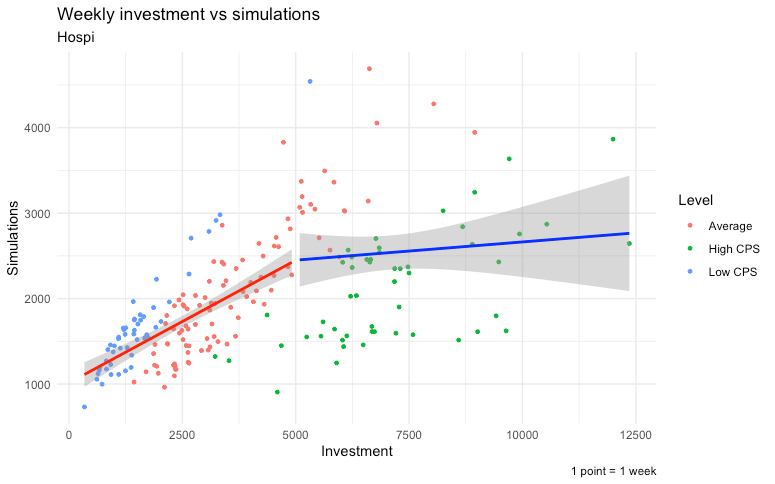
Finding the infliction point of decreasing marginal returns within a channel.
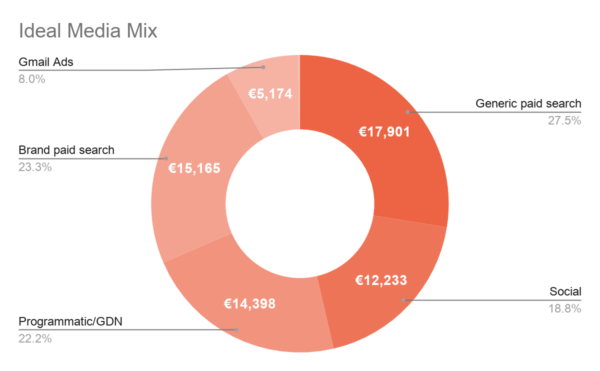
Ideal media mix with % of investment per channel, based on MMM. Just by looking at click-based or even impression-based attribution, Programmatic/GDN would never receive 22% of the total.
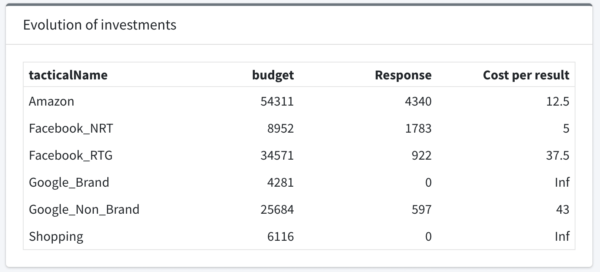
Modelled cost per sale per channel, which allows a comparison to Amazon Ads, a channel that doesn’t show up in your Google Analytics. It also shows that the investment in branded paid search wasn’t incremental. The same thing for Shopping, it wasn’t incremental because most budget went to branded keywords.
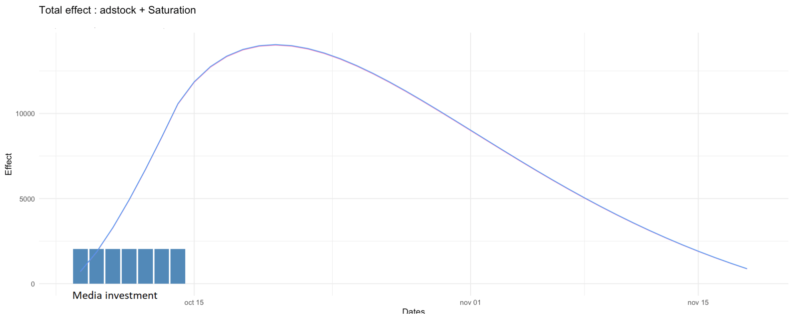
Memorization analysis of the impact of a TV spot on sales. It shows when to ideally launch a new spot: when the effect has faded off.
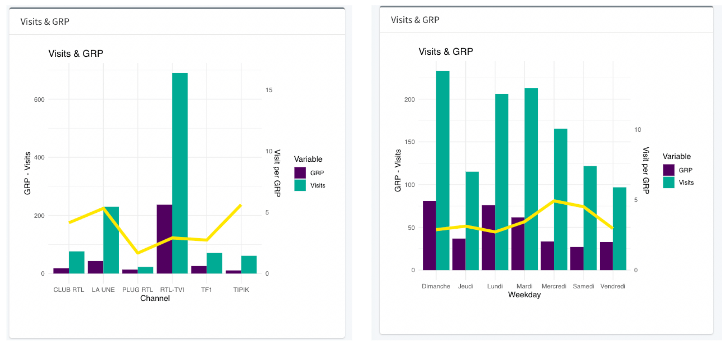
Analysis of contribution to website visits per TV channel. It allows better choices in terms of which TV channels you want to show for the next campaign.
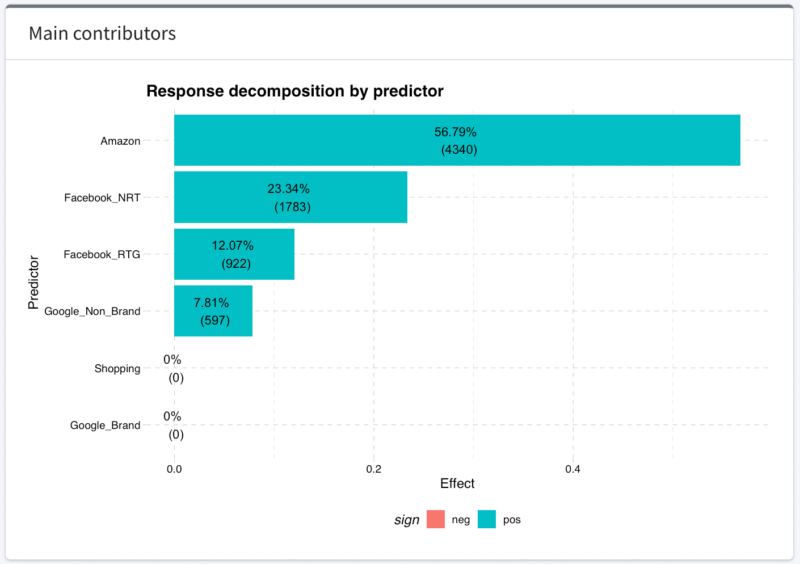
Sales per channel, including Amazon Ads, a channel that doesn’t show up in Google Analytics. Amazon Ads actually had by far the strongest effect on sales.
In short, LYKTA helps clients better answer tactical and strategical marketing questions, like:
- What is the global ROI of paid media investments?
- How many sales would we have if we cut ad spends?
- Which media drives the most sales?
- What is the ROI per channel?
- What is the optimal level of investment?
This doesn’t mean you can throw out your existing marketing measurement stack, though. Measurement tools are complementary.
LYKTA is about uniting these roles, in a single platform.
We’re offering it in 3 packages:
-
- Drive to Web, including analysis and reporting, for a one-off fee of €3 000
- Advanced AB tests, including planning, buying, analysis and reporting, for a one-off fee of €5 000
- The full MMM package, including training sessions, data gathering with your team, the initial model, refreshes of the model based on new data, access to the self-service platform and of course, the 2 packages above, for a yearly fee of €33 000.
Interested? Find out more on www.lykta.be or contact them directly at contact@lykta.be.
Or reach out to us, and we’ll help you get started on this journey.
Ontvang onze inzichten rechtstreeks in je inbox
We duiken regelmatig in actuele onderwerpen op het gebied van digitale marketing en delen onze inzichten graag met jou.